AI could help remove bias from medical research and data
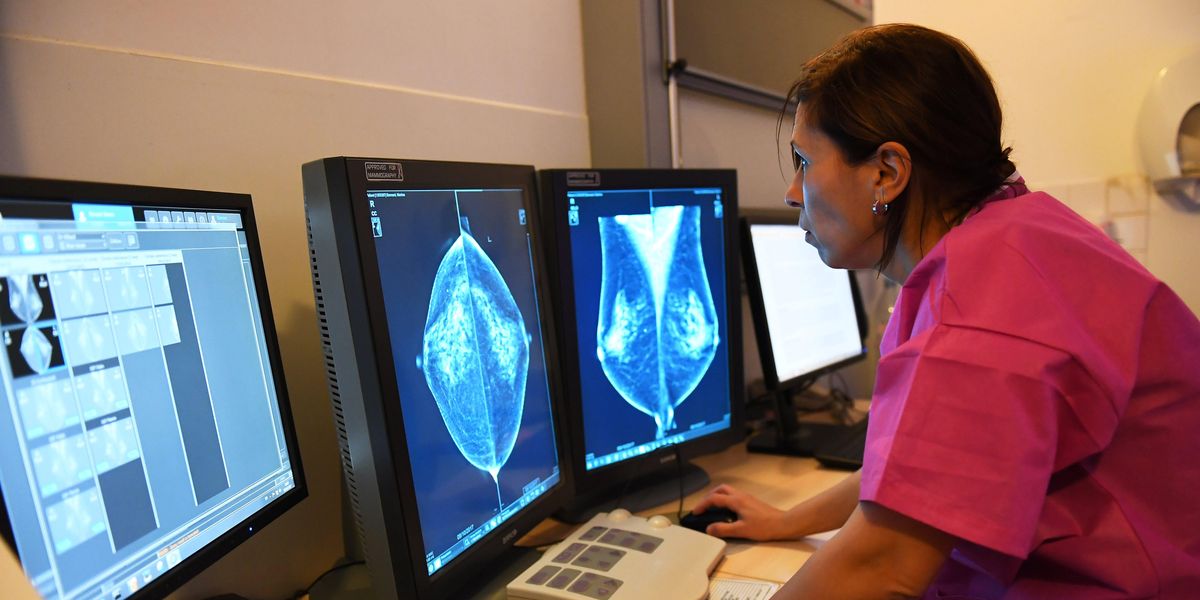
Artificial intelligence can help root out racial bias in health care, but only if the programmers can create the software so it doesn't make the same mistakes people make, like misreading mammograms results, writes Pearl.
This is the second entry in a two-part op-ed series on institutional racism in American medicine.
A little over a year before the coronavirus pandemic reached our shores, the racism problem in U.S. health care was making big headlines.
But it wasn't doctors or nurses being accused of bias. Rather, a study published in Science concluded that a predictive health care algorithm had, itself, discriminated against Black patients.
The story originated with Optum, a subsidiary of insurance giant UnitedHealth Group, which had designed an application to identify high-risk patients with untreated chronic diseases. The company's ultimate goal was to help re-distribute medical resources to those who'd benefit most from added care. And to figure out who was most in need, Optum's algorithm assessed the cost of each patient's past treatments.
Unaccounted for in the algorithm's design was this essential fact: The average Black patient receives $1,800 less per year in total medical care than a white person with the same set of health problems. And, sure enough, when the researchers went back and re-ranked patients by their illnesses (rather than the cost of their care), the percentage of Black patients who should have been enrolled in specialized care programs jumped from 18 percent to 47 percent.
Sign up for The Fulcrum newsletter
Journalists and commentators pinned the blame for racial bias on Optum's algorithm. In reality, technology wasn't the problem. At issue were the doctors who failed to provide sufficient medical care to the Black patients in the first place. Meaning, the data was faulty because humans failed to provide equitable care.
Artificial intelligence and algorithmic approaches can only be as accurate, reliable and helpful as the data they're given. If the human inputs are unreliable, the data will be, as well.
Let's use the identification of breast cancer as an example. As much as one-third of the time, two radiologists looking at the same mammogram will disagree on the diagnosis. Therefore, if AI software were programmed to act like humans, the technology would be wrong one-third of the time.
Instead, AI can store and compare tens of thousands of mammogram images — comparing examples of women with cancer and without — to detect hundreds of subtle differences that humans often overlook. It can remember all those tiny differences when reviewing new mammograms, which is why AI is already estimated to be 10 percent more accurate than the average radiologist.
What AI can't recognize is whether it's being fed biased or incorrect information. Adjusting for bias in research and data aggregation requires that humans acknowledge their faulty assumptions and decisions, and then modify the inputs accordingly.
Correcting these types of errors should be standard practice by now. After all, any research project that seeks funding and publication is required to include an analysis of potential bias, based on the study's participants. As an example, investigators who want to compare people's health in two cities would be required to modify the study's design if they failed to account for major differences in age, education or other factors that might inappropriately tilt the results.
Given how often data is flawed, the possibility of racial bias should be explicitly factored into every AI project. With universities and funding agencies increasingly focused on racial issues in medicine, this expectation has the potential to become routine in the future. Once it is, AI will force researchers to confront bias in health care. As a result, the conclusions and recommendations they provide will be more accurate and equitable.
Thirteen months into the pandemic, Covid-19 continues to kill Black individuals at a rate three times higher than white people. For years, health plans and hospital leaders have talked about the need to address health disparities like these. And yet, despite good intentions, the solutions they put forth always look a lot like the failed efforts of the past.
Addressing systemic racism in medicine requires that we analyze far more data (all at once) than we do today. AI is the perfect application for this task. What we need is a national commitment to use these types of technologies to answer medicine's most urgent questions.
There is no antidote to the problem of racism in medicine. But combining AI with a national commitment to root out bias in health care would be a good start, putting our medical system on a path toward antiracism.
- Institutional racism exists in American health care - The Fulcrum ›
- Political deepfake videos outlawed in California, Texas - The Fulcrum ›
- Can democratic innovations reduce polarization? - The Fulcrum ›
- Avoiding disaster by mandating AI testing - The Fulcrum ›
- Why are Black women becoming the hidden figures in AI? - The Fulcrum ›
- Why ChatGPT’s ‘memory’ will be a health care camechanger - The Fulcrum ›